NeuroAI: A field born from the symbiosis between neuroscience, AI
As the history of this nascent discipline reveals, neuroscience has inspired advances in artificial intelligence, and AI has provided a testing ground for models in neuroscience, accelerating progress in both fields.
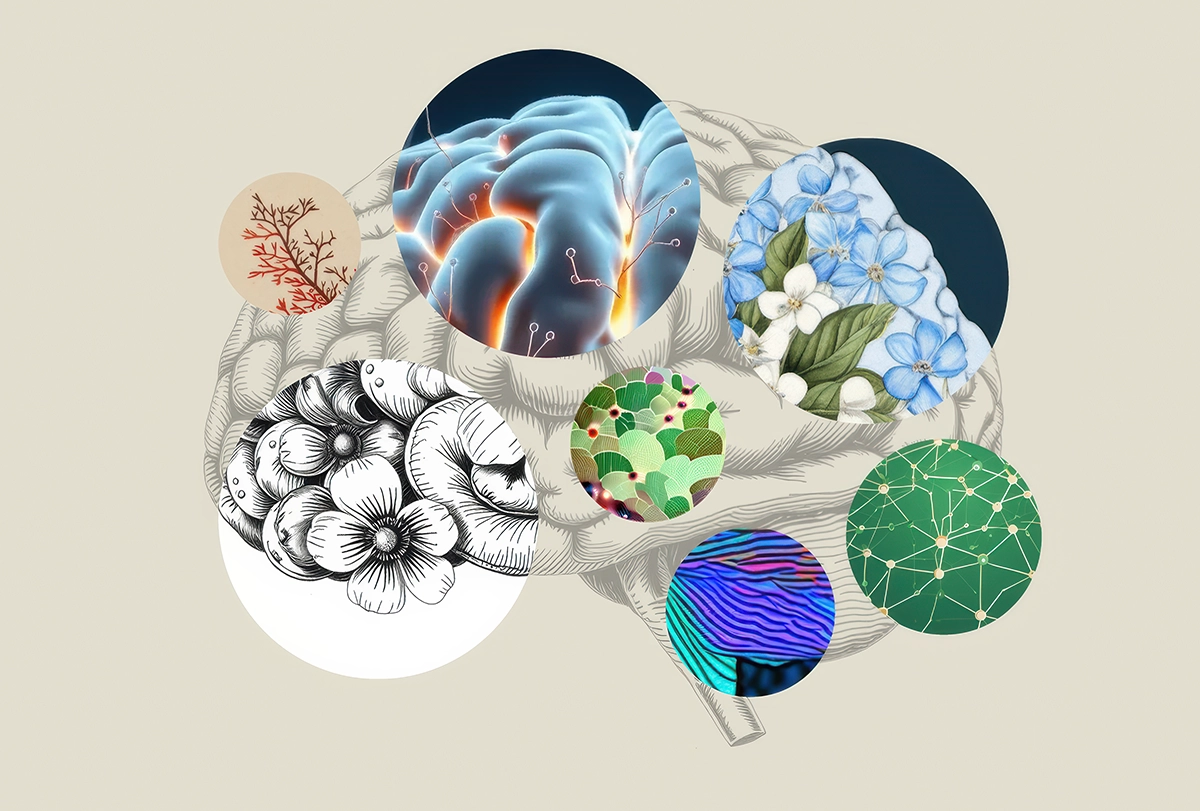
“NeuroAI,” a portmanteau of “neuroscience” and “AI” (artificial intelligence), is on the rise. Almost unheard of until about five years ago, it has now emerged as a “hot” area of research—and the subject of a growing number of workshops, conferences and academic programs, including a BRAIN-Initiative-sponsored workshop that starts tomorrow. The intertwining of these disciplines was almost inevitable. On the one hand, AI aims to replicate intelligent behavior, and the most direct path to this goal is reverse-engineering the brain. On the other hand, neural networks represent the closest model yet of distributed brain-like computation, one that is uniquely capable of solving complex problems.
Here, in the first in an ongoing series of essays on NeuroAI, I will explore the history of the co-evolution of AI and neuroscience, and how the ongoing symbiosis between these fields has created and continues to form a virtuous circle.
The term NeuroAI has no single definition, but it has largely come to refer to two related and partly intertwined research programs. First, it refers to the application of AI to neuroscience—specifically, the use of neural networks as computational models of the brain, which offer a concrete and rigorous way to test otherwise potentially fuzzy hypotheses about how collections of neurons compute. As articulated by Richard Feynman’s famous dictum, “That which I cannot create, I do not understand,” artificial neural networks offer arguably the best model for testing whether we really understand how the brain performs computations.
Second, NeuroAI refers to the adaptation of insights from neuroscience to build better AI systems. The underlying goal of much of AI research is to build artificial systems that can do anything a human can do, and AI engineers have long looked to neuroscience for inspiration. Together, these two research programs—neuroscience inspiring advances in AI, and AI providing a testing ground for models in neuroscience—form a positive feedback loop that has accelerated progress in both fields.
AI can also be used to find structure in large neuroscience datasets or for automating data analysis. The DeepLabCut program, for example, enables researchers to automatically track animal movements. Such applications are important, but they are not qualitatively different from the application of AI to any other field, such as protein folding or image recognition, and so are often not included in the term “NeuroAI.”
T
he connection between neuroscience and computing can be traced to the very foundations of modern computer science. John von Neumann’s seminal 1945 report outlining the first computer architecture, EDVAC, dedicated an entire chapter to discussing whether the proposed system was sufficiently brain-like. Remarkably, the only citation in this document was to Warren McCulloch and Walter Pitts’ 1943 paper, which is widely considered the first paper on neural networks. This early cross-pollination between neuroscience and computer science set the stage for decades of mutual inspiration.Building on these foundations, Frank Rosenblatt introduced the perceptron in 1958. The key breakthrough here was the then-revolutionary idea that neural networks should learn from data rather than being explicitly programmed. The perceptron architecture thereby established synaptic connections as the primary locus of learning in artificial neural networks. (Another of Rosenblatt’s significant achievements was to orchestrate extensive media coverage of this advance, including an article published in The New York Times in 1958 under the headline “Electronic ‘Brain’ Teaches Itself”—the first example of many rounds of the so-called “AI hype cycle.”)
This concept of synaptic plasticity was heavily influenced by neuroscientists, such as Donald Hebb, who in 1949 highlighted the importance of the synapse as the physical locus of learning and memory. Hebbian synapses were central to Hopfield networks, a highly influential neural network model in the 1980s (named for John Hopfield, who, along with Geoffrey Hinton, was awarded the Nobel Prize in Physics this year). Although the perceptron itself did not persist as a viable architecture—Marvin Minsky and Seymour Papert’s 1969 book chapter “Perceptrons” exposed the limitations of single-layer perceptrons and triggered the first “neural network winter”—the core neuroscience-inspired idea that synapses are the plastic elements, or free parameters, of a neural network has remained absolutely central to modern AI.
M
any subsequent success stories of the symbiosis between artificial and biological neural network research have followed. Perhaps the most celebrated is the convolutional neural network (CNN), which powers many of the most successful artificial vision systems today and which was inspired by David Hubel and Torsten Wiesel’s model of the visual cortex more than four decades ago.Another home run is the story of reinforcement learning, which has driven groundbreaking AI achievements, including Google DeepMind’s champion-level game-playing engine AlphaZero. More recently, the concept of “dropout” has gained prominence in artificial neural networks. This technique—in which individual neurons of the artificial network are randomly deactivated during training to prevent overfitting—draws inspiration from the brain’s use of stochastic processes. By mimicking the occasional misfiring of neurons, dropout encourages networks to develop more robust and resilient representations.
It is important to note that the relationship between AI and neuroscience is truly mutualistic and not parasitic. AI has benefited neuroscience as much as neuroscience has benefited AI. For example, artificial neural networks are at the heart of many state-of-the-art models of the visual cortex. The success of these models in solving complex perceptual tasks has led to new hypotheses about how the brain might perform similar computations. Artificial “deep” reinforcement learning, a neuro-inspired algorithm that combines deep neural networks with trial-and-error learning, provides another compelling example. Not only has it driven groundbreaking AI achievements (including AlphaGO, which achieved superhuman performance on the game Go), it has also sparked a better understanding of reward systems in the brain.
The ongoing interplay between neuroscience and AI continues to drive transformative advancements in both fields. As artificial neural network models become more sophisticated, they provide insights into brain function; and as our understanding of brain function increases, it inspires new algorithms that push the boundaries of what artificial systems are capable of. As NeuroAI grows, it promises to accelerate our understanding of intelligence in both biological and artificial realms, reinforcing the deep and lasting connection between these two disciplines. This ongoing essay series will explore these advances and the insights they provide into the brain, as well as some of the practical and ethical issues that they raise.
To learn more about NeuroAI’s future, read my second essay, “What the brain can teach artificial neural networks.”
Recommended reading
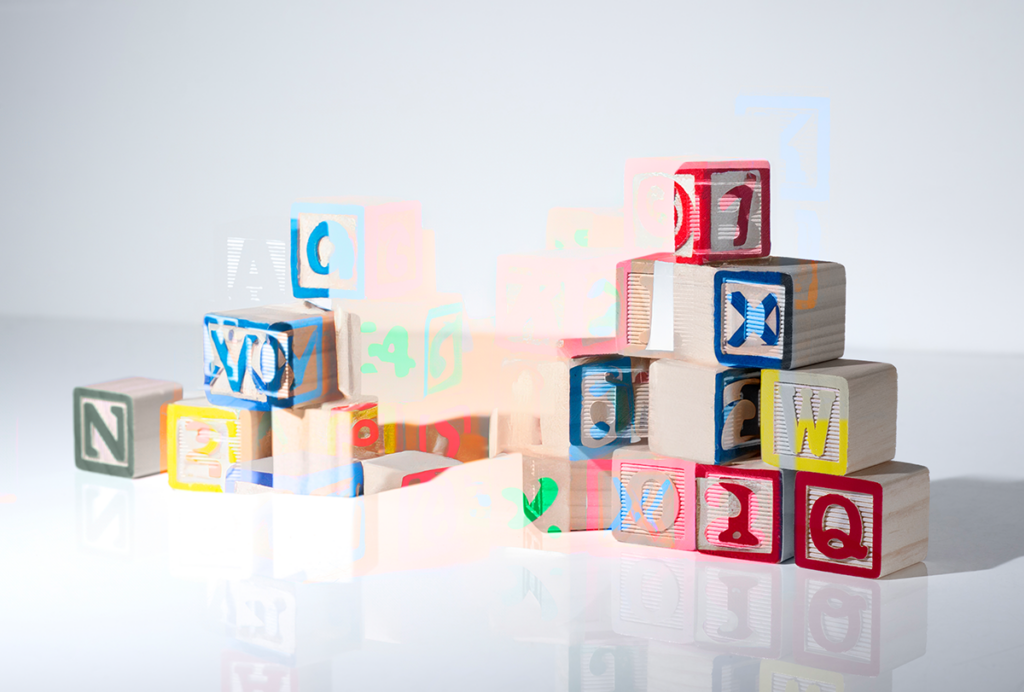
The BabyLM Challenge: In search of more efficient learning algorithms, researchers look to infants
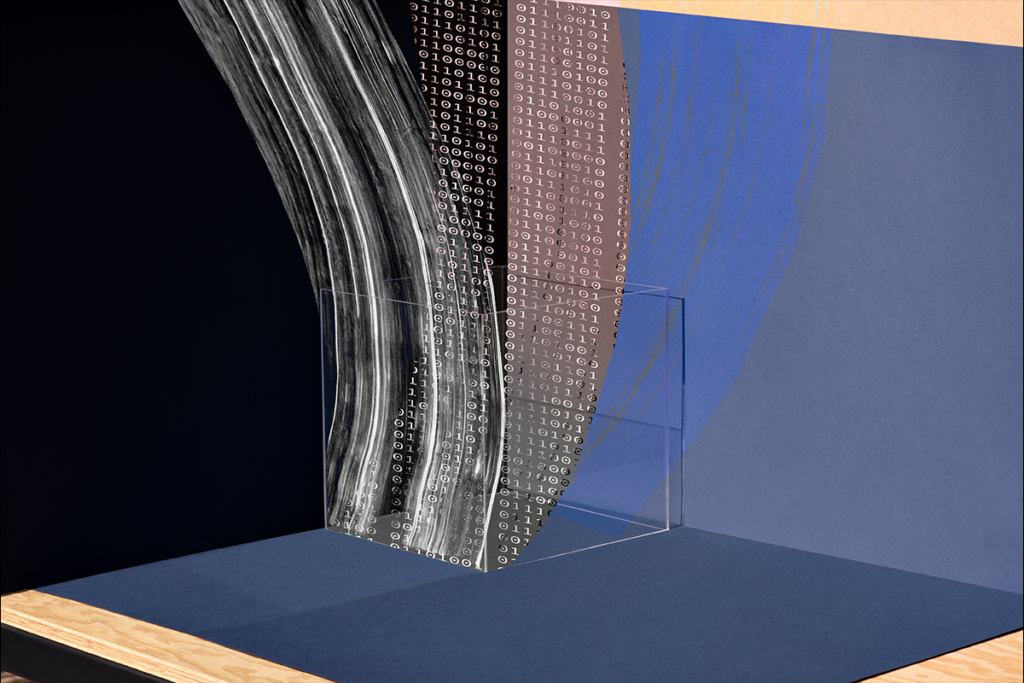
Accepting “the bitter lesson” and embracing the brain’s complexity
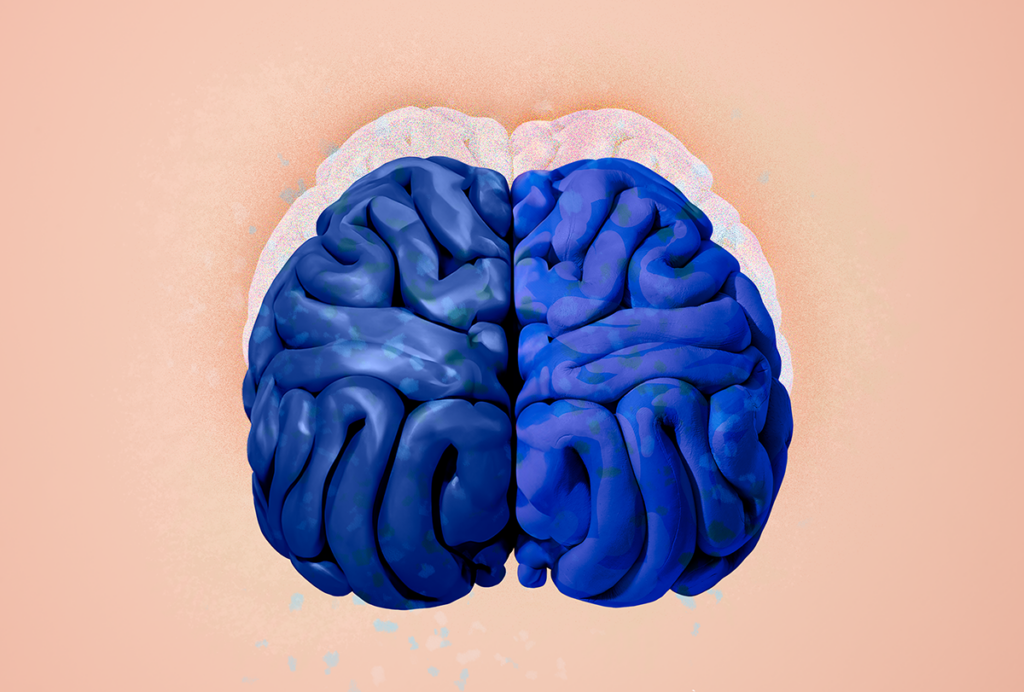
Does the solution to building safe artificial intelligence lie in the brain?
Explore more from The Transmitter
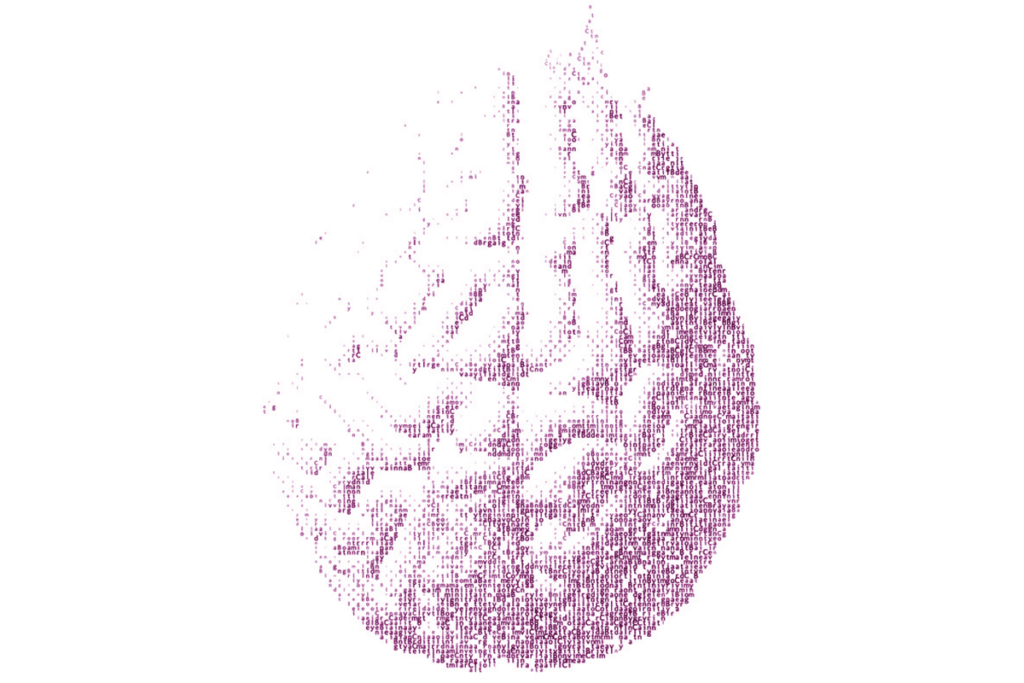
ABCD Study omits gender-identity data from latest release
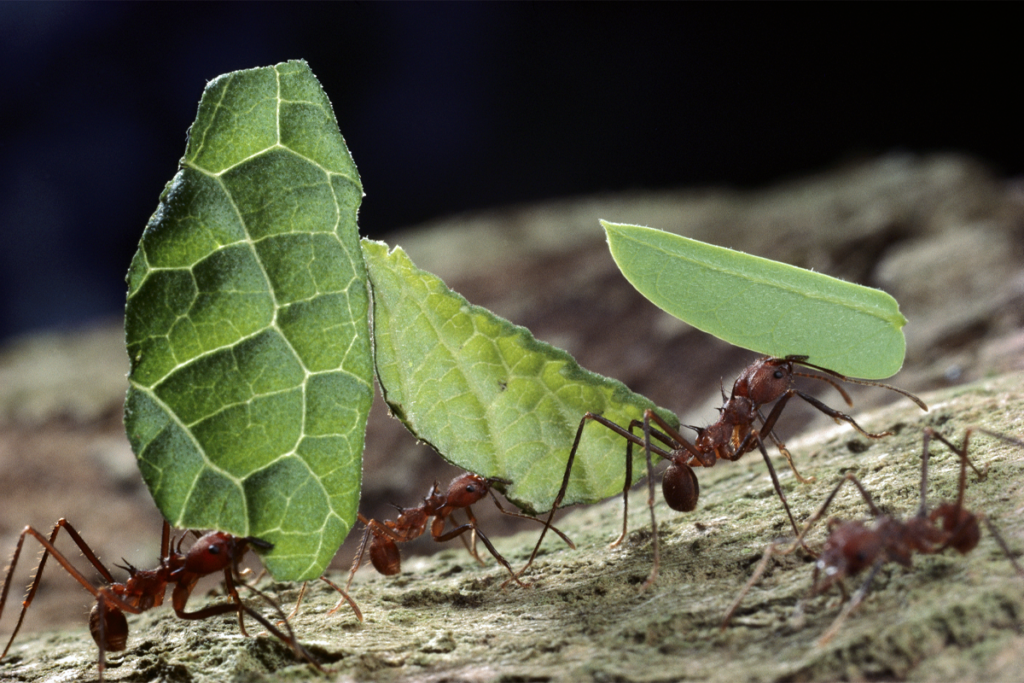
Neuropeptides reprogram social roles in leafcutter ants
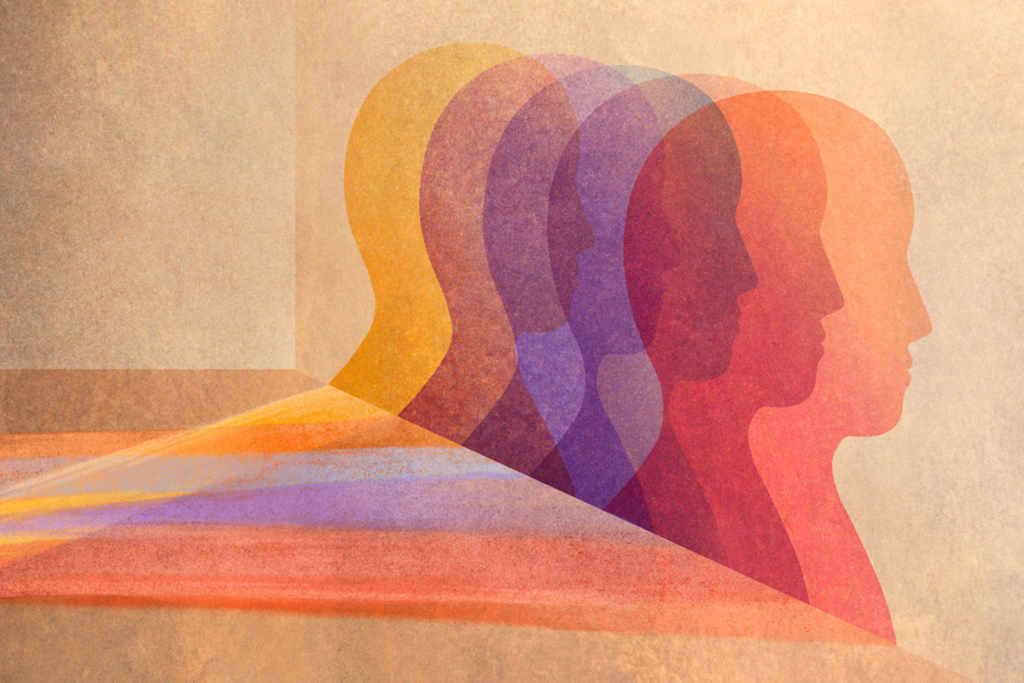